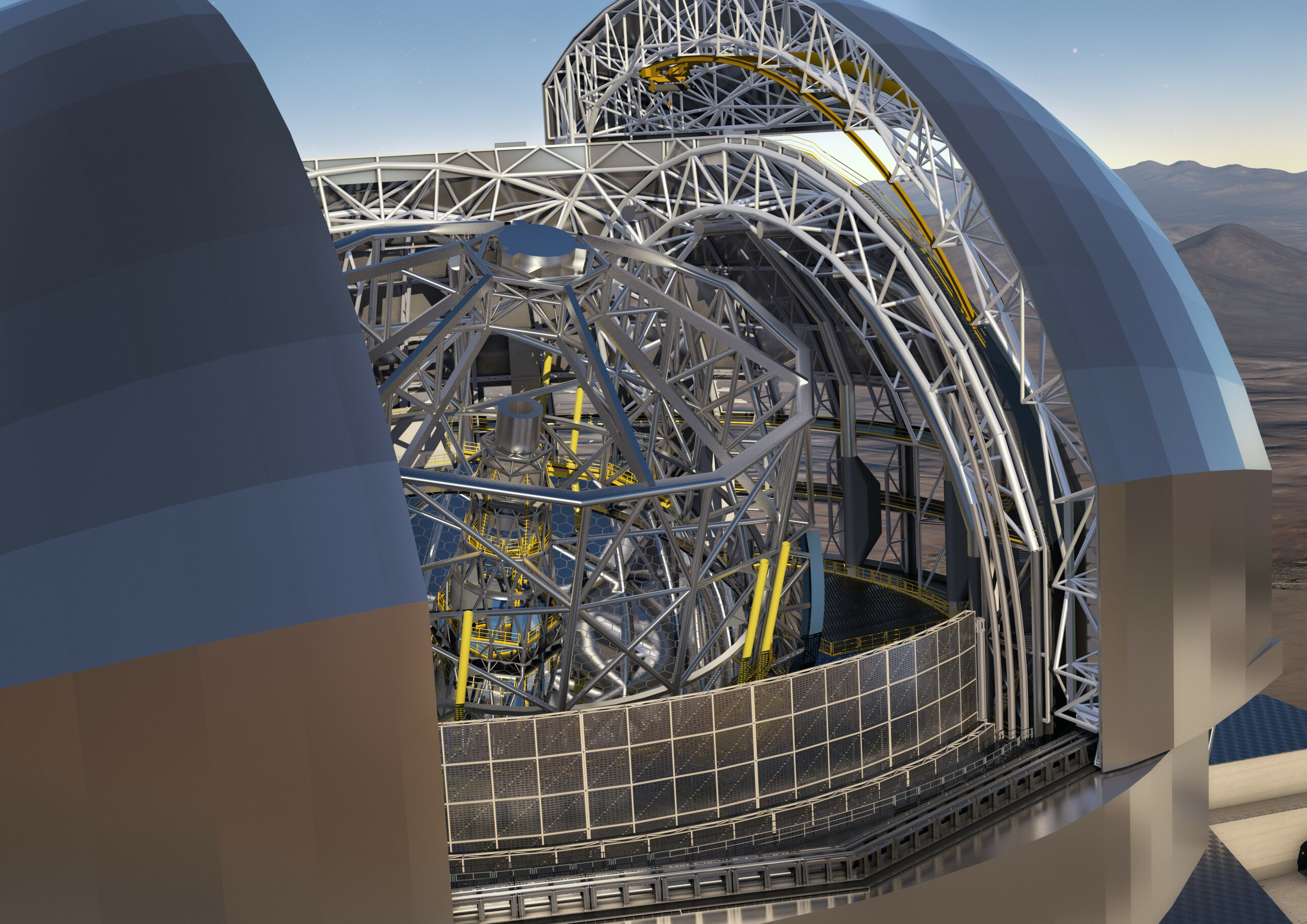
A real-time controller for adaptive optics
Overview
Meet the two technological challenges of real-time control of extreme adaptive optics, and supply large-scale prototypes for the instrumentation of the Very Large Telescope (VLT) and the Extremely Large Telescope (ELT).
Damien GRATADOUR, CR CNRS, LESIA – Observatoire de Paris
Eric THIEBAUT, Astronome, Centre de Recherche Astrophysique de Lyon (CRAL)
Benjamin QUERTIER, IR, Laboratoire d’Astronomie de Bordeaux (LAB)
The first objective of this project is to develop new connectionist architectures, based on modern data-driven machine learning (ML) techniques using deep neural networks (DNN), to solve current phase reconstruction problems for high-angle resolution observations in optical astronomy. Our challenge is to develop new pragmatic techniques that address the non-linearities of optical aberration measurement and compensation systems within an integrated data-driven framework, for the calibration and control of Extreme Adaptive Optics (XAO) systems.
In parallel, this project aims to design, implement and validate a new real-time control (RTC) platform for AO (including hardware and software solutions) providing the means to integrate these new connectionist architectures into the AO control pipeline. This includes: solutions for low-latency, high-speed data ingestion, integration of new computational hardware solutions dedicated to AI task sets, interoperability between task flows of conventional and AI-based control methods, and temporally deterministic implementation in a closed-loop control scenario.
To develop
Our researchs
New generation of cutting-edge technologies for real-time control of extreme adaptive optics
- Develop new low-latency data ingestion strategies, capable of scaling up to the most complex extreme AO systems, and embedding intelligent functionalities (including on-the-fly denoising and/or data compression). The aim is to optimize data transfer to the computation engine, minimizing the footprint of data ingestion on the PSTN latency budget.
- Evaluate data-driven methodologies in OA pipelines and validate converged approaches mixing classical task flows with AI methodologies in the same pipelines. The aim is to assess the compatibility of such hybrid approaches with an advanced RTC platform that would embed AI-dedicated hardware solutions (GPU + IPU) in conjunction with more conventional architectures (CPU + GPU).
- Integrate the above developments into dedicated demonstrators targeting both SPHERE+ and PCS dimensioning. With two different contexts, the targeted level of maturity will be different, with the SPHERE+ demonstrator as a qualification system for subsequent instrument integration, while the PCS demonstrator will be primarily a proof-of-concept in a simulated environment.
Key components of new technologies:
- An intelligent interconnect solution, capable of scaling to the largest extreme OA size, and integrating intelligent AI-based data reduction and compression features
- A new scalable RTC solution for OA, realizing complex converged pipelines mixing DNN and model-based workflows, right through to ELT system sizing.
- One of the ambitions of this project is to bridge the programmability and performance gap between general-purpose hardware gas pedals, new AI application-specific hardware and high-speed, low-latency interconnect solutions to enable the migration of data and tasks to the most sustainable resource in the context of next-generation AO systems.
The consortium
CNRS + REFLEX CES (partenaire non financé)
Lyon (CRAL), Bordeaux (LAB)
… VISIONARY TECHNOLOGIES PAVE THE WAY FOR POWERFUL NEW INSTRUMENTS
- In the short term, our activities lie at the crossroads of engineering and astrophysics, offering numerous opportunities for cross-fertilization between the various fields involved. The collaborative nature of this project is enhanced by the complementary expertise of the research groups involved, which will benefit the post-docs and PhD students assigned to it. In the medium term, the results of this collaboration will be incorporated into ESO’s instrument upgrades and the future roadmap for European astronomical instrumentation.
- An industrial partner REFLEX CES will be able to strengthen and diversify its product ranges, opening up new market opportunities in high-performance computing, a high value-added sector, thanks to in-depth collaboration with a national network of experts.
- Specialized high-performance computing techniques and the management of large volumes of data are transferable to a wide range of scientific fields and industrial sectors.
More projects
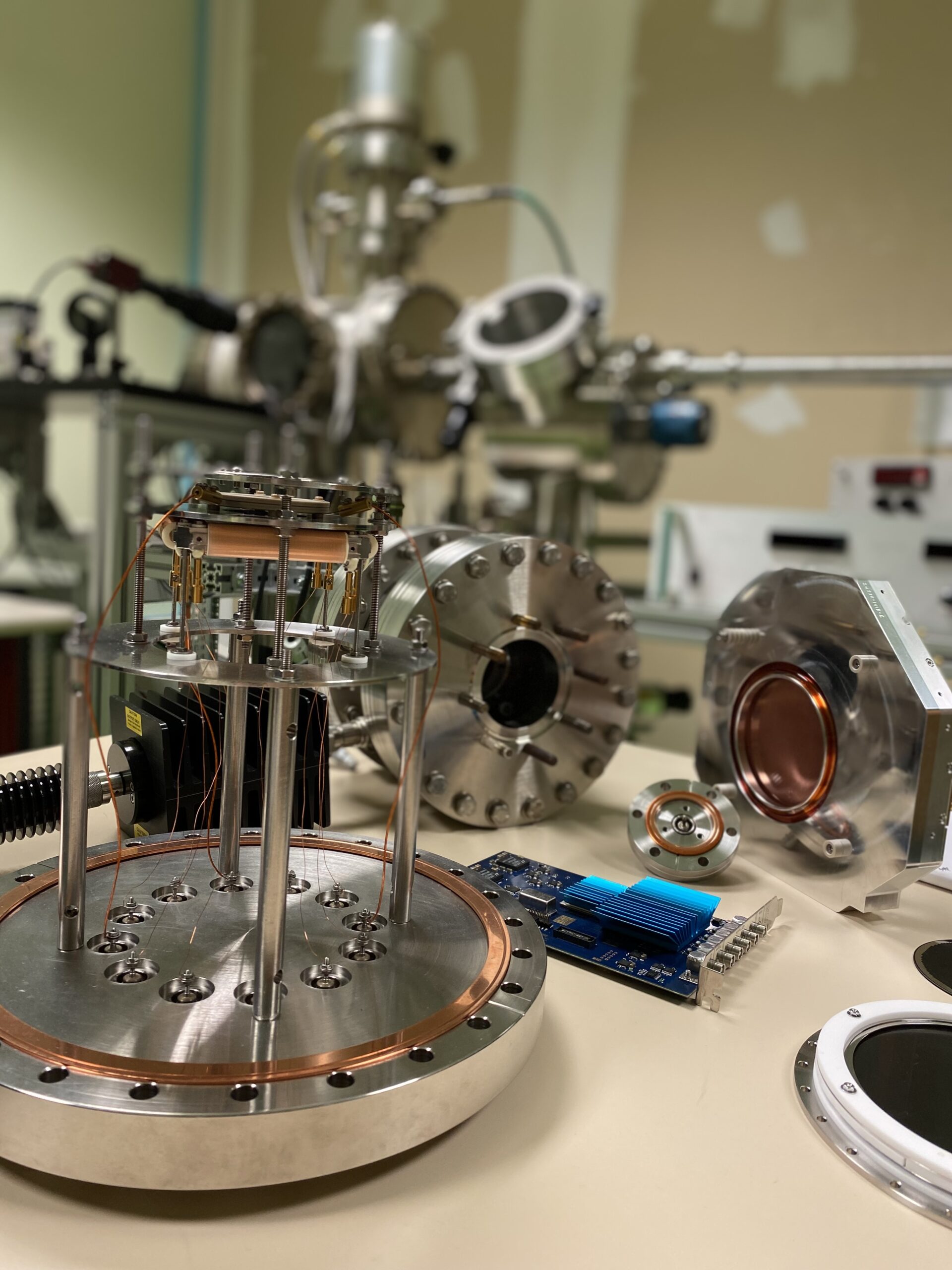
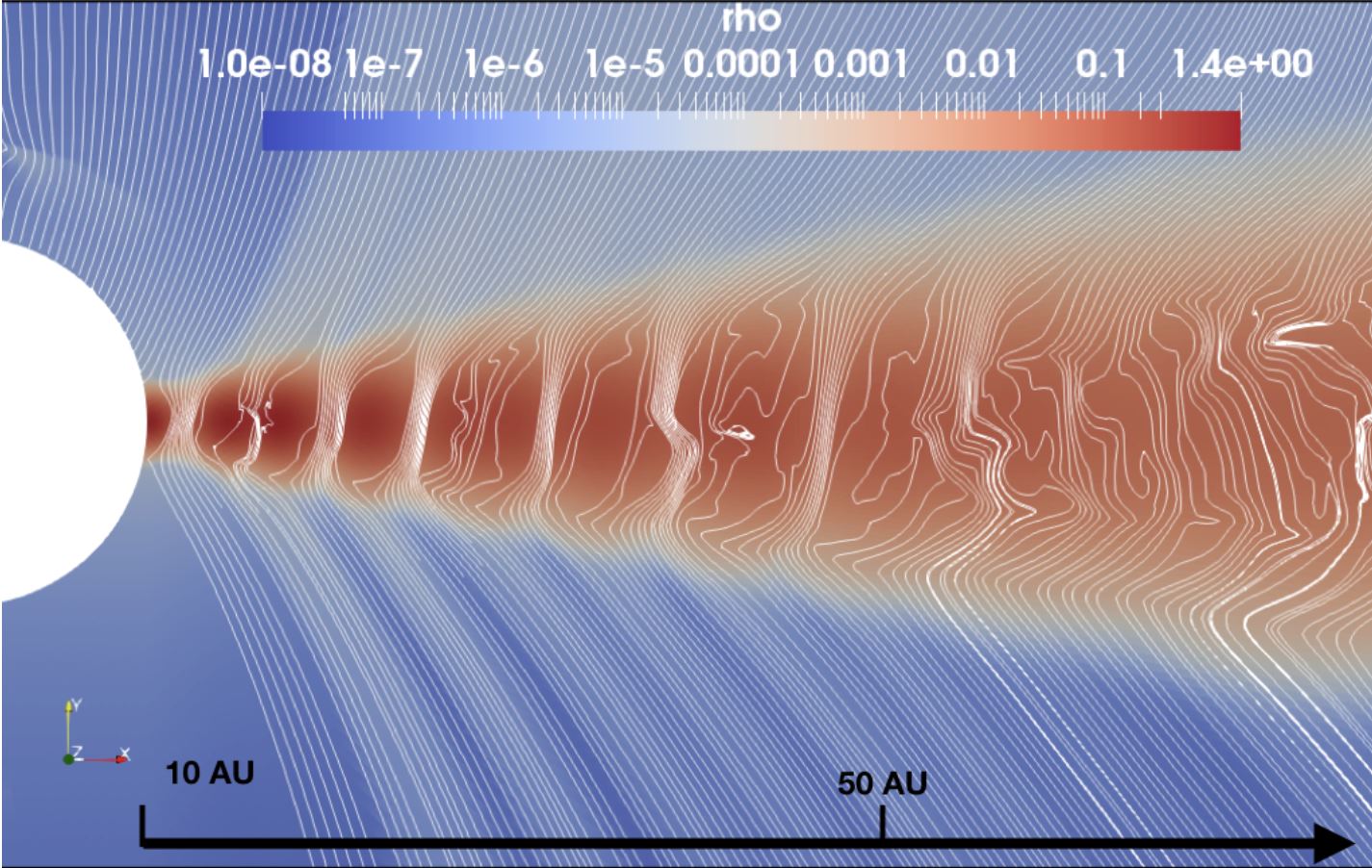