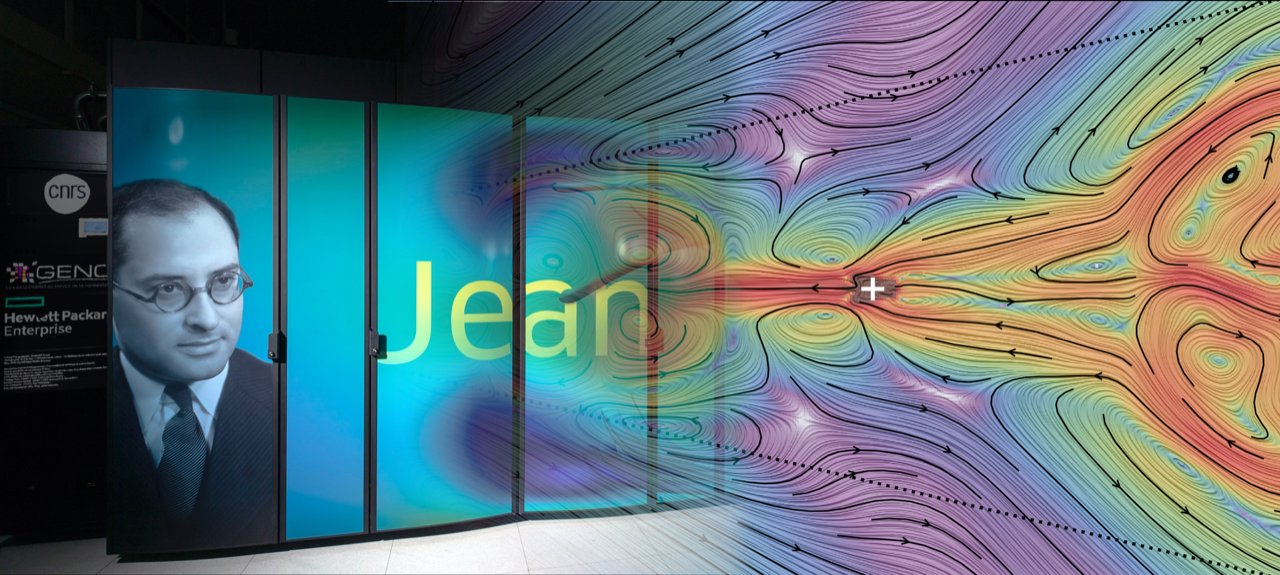
Thematic research :
DIGITAL
Supercomputing/AI simulations and data analysis
The challenge
Make the most of the digital revolution for data analysis and physical modeling.
Overview
The aim is to gain a better understanding of planet formation through the digital revolution. The Origins project will push back the limits of our understanding of the evolution of protoplanetary disks, and improve our ability to search for exoplanets by direct imaging, using developments in Exascale and artificial intelligence.
Scientific
FRAMEWORK
The challenges
The Exascale revolution marks a major breakthrough in computing, with the emergence of new ultra-high-performance machines, driven by international economic, cybersecurity and scientific research challenges (high-performance computing, artificial intelligence). These supercomputers, capable of performing a quintillion floating-point operations per second, push back the limits of computing power thanks to their hardware architecture, which combines central processors (CPUs) and graphics processors (GPUs). This combination optimizes both performance and environmental impact, reducing energy consumption and carbon emissions. We need to develop algorithms capable of fully exploiting these new architectures, and train a new generation of scientists in these new technologies. The challenge of the PEPR ORIGINS project is to work with the scientific community to develop our digital tools in the direction of Exascale and artificial intelligence.
Scientific framework
Modern theoretical planetology relies on multi-scale and multi-physics magnetohydrodynamic numerical simulations, essential for understanding the formation of stars and their disks, the cradles of planetary formation. We will be developing innovative physics modules in the Exascale-targeted codes DYABLO, IDEFIX and SHAMROCK to deal with the evolution of magnetized fluids and the interaction between different fluids, and to include Lagrangian tracers. Modern observational planetology relies on artificial intelligence for real-time control of adaptive optics, hyper-spectral image analysis and multi-variate data analysis. We will be developing innovative Artificial Intelligence methods capable of automatically coping with the diversity of very large volumes of data and changes in acquisition conditions, with substantial gains to be made.
Future instruments
Modeling and observing exoplanet formation processes requires technological leaps in the numerical methods employed. The first project will develop a complete MHD code with all realistic physics modules, adapted to run on the new Exascale GPU/CPU environment; the second project will develop unsupervised artificial intelligence methods for analyzing noisy data, in particular to detect exoplanets in hyper-spectral images. The last two projects will develop unsupervised algorithms for real-time control of the adaptive optics needed for these detections, and for scheduling observations to take account of scientific priorities and sky quality constraints.
Axis managers :
Maud LANGLOIS : maud.langlois@univ-lyon1.fr
Guillaume LAIBE : glaibe@ens-lyon.fr